AI in healthcare data management: A comprehensive guide
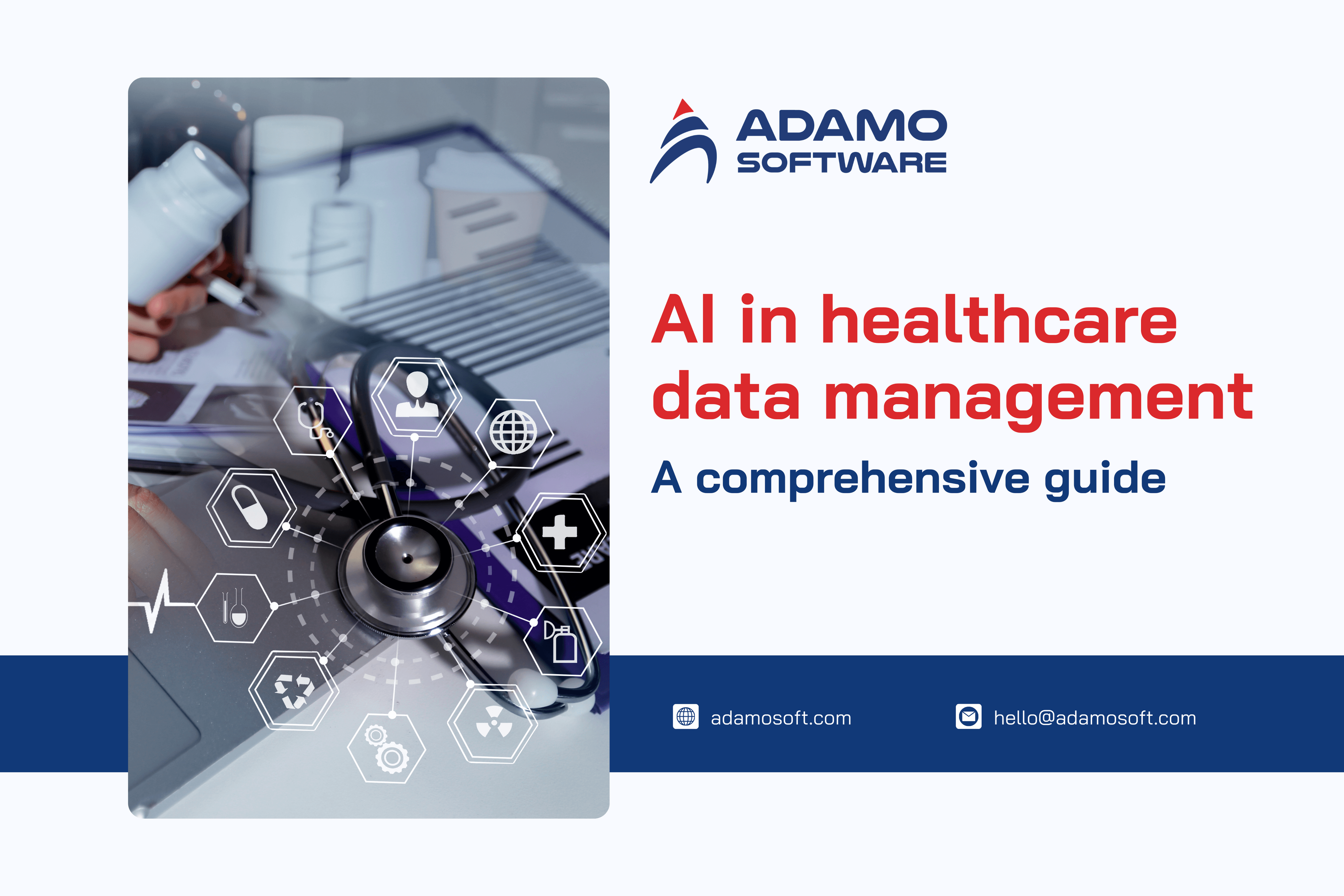
Step into the future! Explore 2025’s AI in healthcare data management revolution, where smarter systems meet life-saving precision.
The healthcare industry benefits from AI in healthcare data management solutions because it brings innovative answers to address business challenges of growing size. The growing surge of patient data has made AI essential for improving healthcare operations, decision-making clarity, and better patient results. AI in healthcare data management enhances healthcare organizations by automating data entry and uncovering critical insights from large healthcare databases.
This article explores essential information relevant to 2025 while demonstrating AI’s role in fixing current operational weaknesses. It also discusses the establishment of an individualized healthcare network with enhanced efficiency.
I. The overview of AI in healthcare data management
AI in healthcare data management achieves vital patient record management optimization, processing, and usage capabilities as a healthcare data management tool. Healthcare organizations process significant daily data based on the U.S. clinical trial numbers from 2024, amounting to 6,000 trials.
Healthcare data management operations become more efficient through AI in healthcare data management because it decreases medical errors and creates better patient results. AI solutions could reduce 86% of errors among healthcare staff, potentially saving 250,000 lives by 2030.
AI-led healthcare data management relies on predictive analytics to study electronic health records, medical imaging, and genetic data to help detect diseases early. This approach also aids in planning treatments and tracking recovery. AI also optimizes administrative processes through healthcare data management, leading to a 79.2% reduction in emergency room visits and hospital admissions.
AI technologies such as machine learning and natural language processing enhance healthcare data management by improving clinical data processing. This supports providers in offering individualized care through secure systems that operate within regulatory frameworks.
II. The challenges of Traditional Healthcare Data Management
1. Data volume
Traditional healthcare data management faces substantial difficulties due to its enormous, constantly expanding data pool. Multiple data formats generate this data collection, including numerical entries and codes alongside physician notes and medical images, which are unstructured data types. Conventional systems struggle to manage large, two-sided data sets effectively, as they do not adapt well to growing data volumes.
2. Data accuracy
Traditional healthcare data management faces critical difficulties in maintaining accurate data, which is one of its main challenges. Errors in manual data management procedures result in damaging effects such as incorrect medical diagnoses, inappropriate treatment choices, and incorrect financial data reporting. The occurrence of inaccurate data processes directly works against both medical care quality and operational effectiveness.
3. Data integration
Standard healthcare data management experiences major difficulties uniting data sources into one cohesive system. Healthcare providers maintain separate data systems and databases, which prevents them from creating one unified, complete information overview. The multiple data sources create analysis delays, which prevent essential decisions and reduce the overall power of analytical efforts.
4. Data security
Protecting health data remains a continuous issue within traditional hospital data management systems. Healthcare organizations encounter great difficulties when safeguarding patient information and following HIPAA laws. Due to weak security protocols, traditional data management systems expose organizations to greater risks of data breaches and compliance issues.
The urgent need for innovative solutions became apparent through these healthcare challenges, which AI in healthcare data management can now address effectively.
III. How AI addresses hurdles in Healthcare Data Management
Healthcare organizations function under intensified requirements for the modernization of systems, steadfast delivery of quality care, and secure data protection. AI in healthcare data management transforms operations by enhancing efficiency, accuracy, and patient care while addressing challenges like data integration, security, and volume.
1. AI-powered virtual assistants and generative chatbots
Medical staff experiences burnout and response delays when handling patient interactions and repetitive tasks. AI in healthcare data management uses virtual assistants with patient-driven programs to maintain round-the-clock appointment scheduling, health question response, and medication scheduling capabilities. AI systems evaluate spoken words to create individualized patient interactions, enabling caregivers to give their attention to essential medical requirements.
Existing AI chatbots support patient-specific healthcare through their ability to review medical information, patient choices, and communication history for customized responses. AI uses stored patient information to provide personalized health awareness tools, appointment suggestions, and symptom-adapted advice. This enables healthcare providers to serve many patients while upholding medical quality efficiently.
2. Streamlining medical records with AI
Multiple system fragmentation within medical records prevents efficient cooperation among medical practitioners during treatment provision. AI in healthcare data management enhances medical data handling by integrating diverse data sources, such as handwritten notes, test results, and interpretive images, into a streamlined system. The tools transform unorganized data into searchable formats, improving care outcomes and operational performance during consultations and emergencies.
3. Integrating patient data for holistic care
Medical personnel often encounter difficulties when merging different forms of patient-related data. The management of healthcare data using AI applications solves this problem through:
- Centralize data sources: Health institutions can enhance data unification by integrating electronic health records with wearable device data and genetic information.
- Build comprehensive patient profiles: Healthcare professionals should build patient profiles that accumulate extensive medical histories to discover developmental patterns across time.
- Promote proactive care: The platform uses real-time monitoring to help medical staff discover medication interactions and allergic reactions and inform them about impending complications.
- Personalize treatment plan: By consolidating aggregated data, healthcare providers can create specialized treatment interventions that reflect individuals’ medical records and genetic composition over time.
Through this integrative approach, healthcare teams convert disordered patient data into valuable insights, leading them to provide safer, coordinated, patient-centered care.
4. Extracting actionable medical insights
Medical practitioners may stumble upon various obstacles when extracting valuable information from large clinical data databases. Detecting underlying patterns in patient data through AI in healthcare data management removes this challenge.
Healthcare organizations benefit from these capabilities to notice early warning signs of declining health and identify patients needing preventive care. Additionally, they can receive treatment recommendations based on historical data. AI’s assessment of complex data enables healthcare teams to draw better decisions that lead to improved patient results.
5. Enhancing medical coding and billing efficiency
The financial processes in healthcare often struggle due to challenges in medical coding and billing. AI in healthcare data management simplifies medical billing by reviewing health records, assigning billing codes, and performing payer requirement checks. The systems utilize current coding patterns, which leads to fewer mistakes during payment processes and a consistent income stream. Process advancements like automated code recommendations and instant compliance checks enhance efficiency.
6. Simplifying insurance claim processing
Insurance claim handling becomes complex because of uncompleted documentation, policies that do not match, and the need for extended claim cycles. AI in healthcare data management streamlines claims processing with automated document scanning and verification of insurance coverage before submission. The advanced implementation of this approach leads to higher new claim acceptance rates and faster payment times.
Counterintuitive analysis enables better claims processing transparency by generating uniform audit trails documenting the entire submission and adjudication sequence. The trails deliver live system information that detects inconsistencies and minimizes conflicts to build strong relations among providers and insurers. The system operations benefit from AI, which performs data orchestration functions and routine tasks. At the same time, human teams address complex disagreements and exceptions to handle policy matters, creating an overall efficient system.
7. Tackling insurance denials with AI
Handling an insurance claim denials burdensome administrative staff and further delays revenue cycle completion. AI in healthcare data management analyzes past rejected claims to identify patterns and predict future denials, improving the overall claims process efficiency. Healthcare professionals utilize automated systems to create denial appeal reports, providing evidence and tracking progress to identify and improve procedural weaknesses.
AI enables healthcare providers to handle complex tasks that need human involvement while maintaining continuous revenue flow through the automation of manual operations. The system also improves operational quality and reduces economic loss from denied claims.
8. Streamlining revenue cycle management (RCM)
Revenue cycle management (RCM) is a leading healthcare administration challenge because manual processes cause inefficiencies. Checking insurance coverage requires extensive time and the process of getting procedure approvals, while manual claim submission frequently results in errors and delays.
The administrative workload increases when healthcare organizations handle insurance denials because preparing appeals requires substantial time and financial resources. Additionally, the medical coding process and payment collection require intense effort due to lengthy workflows. This impacts healthcare speed and increases stress for staff.
AI in healthcare data management provides transformative solutions to overcome these operational inefficiencies. AI integrates diverse healthcare information into electronic health record (EHR) systems, which offer patients access to complete historical medical records. Staff members receive increased time to perform critical duties because administrative tasks, including appointment scheduling and post-operative reminder distribution, now operate through automation.
AI in healthcare data management produces strategic medical information from complicated data collections to assist doctors with diagnosis and treatment preparation. Healthcare patterns and trends AI discover enable medical staff to reach evidence-based clinical solutions.
The drug discovery industry also benefits from AI in healthcare data management, using it to manage significant data volumes, enhance findings development, assess regulatory requirements, and predict therapeutic outcomes.
9. Cutting operational costs with AI
Inefficiencies in healthcare operations, particularly in managing medical records, exchanging data, and handling administrative tasks, lead to higher resource expenses and overall costs. Medical records management often becomes complicated because healthcare organizations must balance rapid access for approved staff members with HIPAA security regulations. Managing records manually and maintaining them throughout their lifecycle can also lead to higher costs and increase the risk of penalties for non-compliance.
The exchange of healthcare data introduces difficulties because healthcare organizations need to merge different types of patient data effectively. This includes demographic information, insurance records, and medical background. Traditional methods of processing data struggle to provide accurate results and quick access to information, which means they often need a lot of staff to manage them. Therefore, the required manual process for appointment scheduling, inter-system referral transfer, and lab integration requirements affects patient and staff experiences.
AI in healthcare data management presents new methods that tackle these challenges. AI centralizes protected medical records, secures them to maintain HIPAA compliance, and allows authorized personnel to obtain information easily. Records management benefits from automation because it eliminates the need for human handling, thus lowering potential risks.
Healthcare data exchange becomes simpler through AI in healthcare data management, which merges multiple data types, facilitates accurate and quick information retrieval, and reduces administrative burdens. The patient experience improves with AI in healthcare data management, which helps automate appointment scheduling and simplifies information retrieval, allowing patients to be more involved in managing their healthcare.
This solution enhances patient care by streamlining referrals, improving lab connections, and strengthening the overall patient experience. AI in healthcare data management also assists healthcare staff by managing provider credentialing and data organization, freeing up more time for clinical activities.
Overall, AI technologies enhance healthcare organizations’ ability to sustain operational success through improved workflow optimization, resulting in better patient care delivery.
Read more: Computer Vision in Healthcare: What it is, real hospital use-cases, and limitations
Explore Our Tailor-made Software Development Solutions
We are confident in providing end-to-end software development services from fully-functioned prototype to design, MVP development and deployment.
IV. Tips to build an effective, trusted AI-augmented healthcare data management system
1. Addressing data fragmentation
Several unconnected healthcare data sources produce electronic health records (EHRs), including wearable devices and telemedicine platforms with consumer genomics data. A disintegrated data structure generates operational troubles because independent datasets block complete examination and process optimization. AI-based data management systems function as a harmonizing agent, creating one interconnected, interoperable system from multiple separate data sources.
Integrating diverse data types, such as patient wearables and medical records, enables AI to provide comprehensive, up-to-date patient evaluations. The achievable interoperability between multiple data sources leads to decreased clinical mistakes, better patient results, and enhanced administrative choices. AI automation tools streamline operations by merging data for different systems, including laboratories, pharmacies, and mini payments.
2. Automating compliance
The traditional manual compliance monitoring system takes too long and frequently produces errors. The efficiency problems concerning HIPAA audits and real-time access tracking get resolved through AI systems, which spot access irregularities and patient database mismatches. Healthcare teams focus on quality care delivery while this system minimizes legal risks.
Current compliance methods that prove inadequate open organizations to noncompliance breaches. AI solutions enhance security by creating permanent audit records. They quickly identify non-compliant data-sharing activities, helping to mitigate vulnerabilities. High-level monitoring software helps medical organizations follow GDPR CCPA regulations alongside other rules by offering continuous privacy protection.
Also read: Machine Learning in Healthcare: What is it, benefits & use cases
3. Preventing errors in data entry
Medical mistakes in recorded data generate incorrect diagnosis results and billing complications that damage patient trust and reduce operational effectiveness. AI in healthcare data management ensures accurate data by identifying inconsistencies in newly entered information against established historical patterns. This process helps make reliable clinical decision-making possible because it decreases the number of denied claims and improves data accuracy.
AI automates error correction for lab code mistakes and merging file duplication, which enhances manual labor efficiency while preserving excellent data clarity. AI text analysis also analyzes doctors’ handwriting to decrease the number of typing errors that plagued previous medical technology systems.
4. Catching health issues early
Health problem detection delays escalate healthcare costs while adversely affecting patient health outcomes. Medical professionals obtain proactive capabilities from AI predictions through continuous patient monitoring, pattern detection in laboratory tests, and smart device information consolidation.
The tools recognize early warning signs for sepsis twelve hours before, predicting hospital re-entry and alerting doctors about drug safety concerns. AI enhances healthcare delivery by revealing treatment performance statistics and improving staffing strategies. This shift moves the system from treatment-based services to proactive prevention while minimizing spending.
5. Ensuring safe system transitions
Medical institutions face massive operational interruptions and substantial financial costs when switching their medical record systems. Data corruption or loss becomes a common issue during the changeover process.
AI in healthcare data management facilitates smooth system transitions by ensuring accurate data transfers and resolving terminology differences, like “HTN” versus “hypertension.” Such a system ensures patient histories stay complete while system downtimes decrease, and deployment times shorten. Data protection through system updates by AI helps stakeholders feel confident and minimizes financial losses accompanying platform modifications.
6. Building transparent AI decisions
Medical professionals require trust in AI systems through clear and detailed explanations of the reasoning behind the advice or alert messages received. AI in healthcare data management supports transparency by revealing decision-making methods during risk determination and treatment suggestion processes. Staff should be able to override AI decisions, fostering collaboration and ensuring AI is a supportive tool, not a replacement.
7. Securing scalable healthcare data systems
Healthcare data systems must establish dual priorities of security strength and flexible growth capabilities. AI in healthcare data management uses advanced encryption to create complete data protection for stored information and information in transit. The healthcare system completely protects user identity through multi-factor authentication and permission tier systems with automatic ongoing monitoring and threat removal.
The system’s fundamental requirement centers on compliance through HIPAA adherence and SOC 2 certification oversight for operational reliability validation. Additionally, HITRUST provides standards proving that the system effectively handles risks so healthcare providers maintain confidence in their data security and privacy.
The AI systems automatically modify their processing capabilities to adapt to peak healthcare emergencies. Optimal performance comes from workload distribution between several locations, while redundant data backup systems protect information access. The scalability and security features enable healthcare organizations to maintain patient information accessibility during changing requirements.
8. Advancing with self-learning AI
The quickly changing medical sector renders static AI models obsolete at a fast pace. Self-learning AI systems solve this issue through their ability to update CDC guidelines and emerging cancer treatments. The systems update their prediction models through new care practices to maintain accuracy while staying relevant during time changes.
Hospitals using self-learning AI systems and AI in healthcare data management develop tools capable of enduring medical advances while gaining knowledge independently. Such systems improve prediction accuracy and operational efficiency by aligning with standard healthcare practices.
Healthcare organizations can use AI in data management to reduce operational issues. This approach also enhances the quality of medical care. Administrative teams must resolve record fragmentation and compliance issues as they demonstrate measurable financial return on investment. Also, A user-friendly design with technological complexity fosters trust in systems essential for daily medical operations.
V. How Adamo Software applies AI in Healthcare Data Management Systems
The healthcare data management systems developed by Adamo Software use AI in healthcare data management to enhance operational efficiency for better results. Our company’s solutions utilize AI analytics tools to handle large datasets with operational efficiency. Predictive data insights enable early detection of medical issues and improve clinical decision-making.
Healthcare providers benefit from Adamo’s expertise because they can optimize work processes while reducing manual mistakes and boosting data protection measures. Contact us now to transform your healthcare systems with our advanced AI-based data management solutions!