Machine Learning in Healthcare: What is it, benefits & use cases
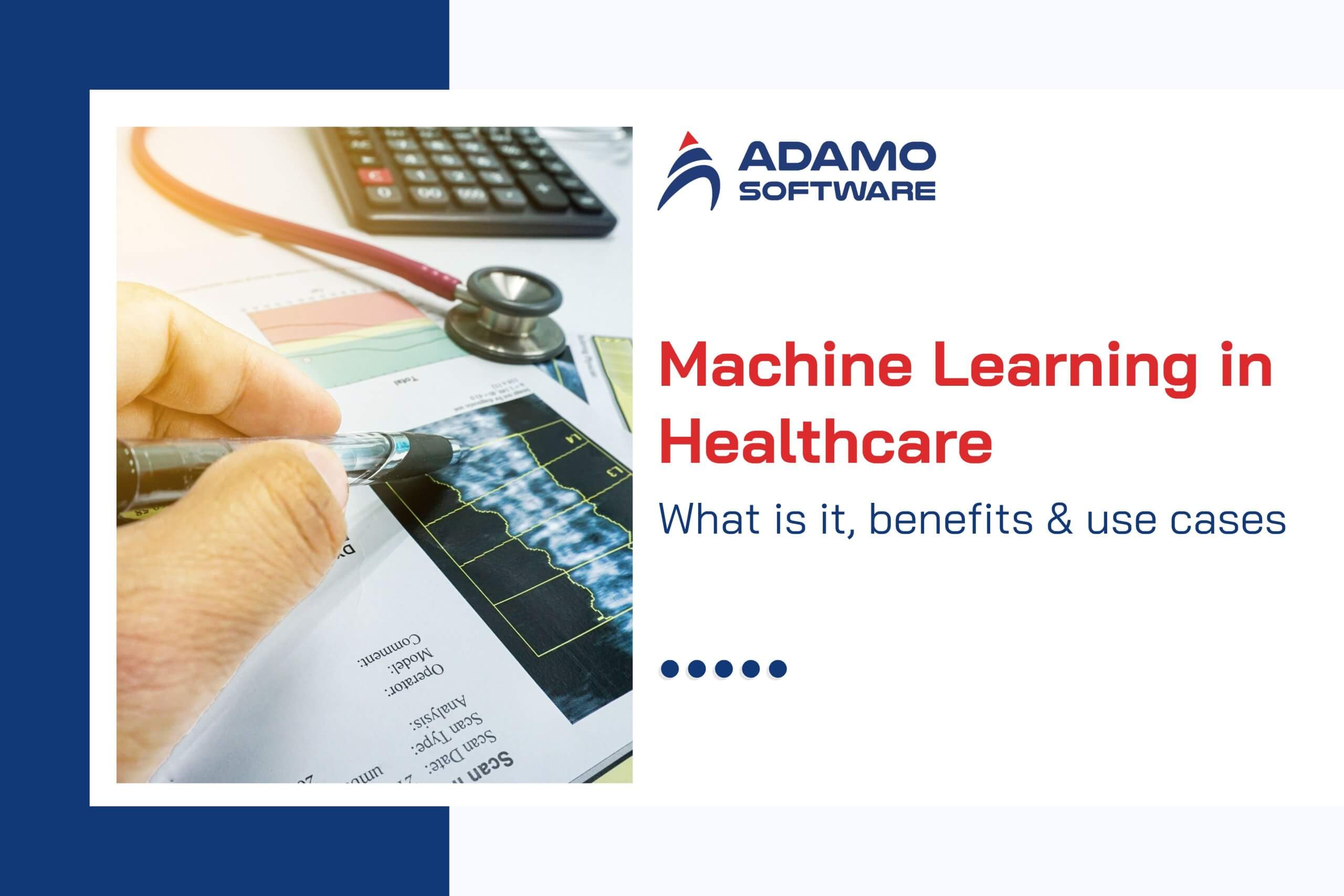
Adopting machine learning is essential in the healthcare industry. Let’s explore machine learning in healthcare and its application with Adamo Software!
In today’s digital era, many industries have applied modern technologies to enhance operational efficiency. Healthcare sectors are also among them. With its profound impact, machine learning is an essential tool in the healthcare industry. It helps to foster precision, efficiency, and advancements in all aspects of patient care. Machine learning in healthcare operates through advanced algorithms and insightful data analysis. This enables healthcare organizations to address various challenges, enhance patient care, and develop sustainable solutions. Let’s explore machine learning in healthcare and its application with Adamo Software!
Through this blog post, you will know the definitions of machine learning in healthcare and how it is different from AI. Besides, you will also get some insights about the benefits and challenges of machine learning in healthcare. Additionally, Adamo Software also discusses the best use cases of machine learning in healthcare. All information has been thoroughly researched and updated to the latest trends. So, let’s read our post and find some useful information!
I. What is Machine Learning in Healthcare?
If you want to know more thoroughly about machine learning in healthcare, you may first find out the definition of machine learning. ML is an AI branch allowing computers to learn from data or interactions to improve performance. With machine learning, the healthcare facilities’ systems can anticipate and act autonomously, without specific programming.
Machine learning in healthcare is getting more popular. It involves analyzing complex medical data with advanced algorithms and computational models. With machine learning, you can get some insights and disease predictions that were hard to achieve in the past. ML’s ability to identify patterns and trends in vast datasets empowers healthcare providers to deliver more precise, efficient, and personalized care.
Machine learning is applied in various healthcare fields, including diagnostics, treatment planning, drug development, and administrative processes. This shows that machine learning is essential for the healthcare industry. Let’s explore some outstanding features of machine learning in healthcare with Adamo Software!
1. Mining massive medical data
Machine learning excels at rapidly and accurately processing massive structured and unstructured data. They include EHRs, medical images, genomic data, etc. This allows for earlier disease detection, patient outcome prediction, and enhanced operational efficiency. Medical information spans many different formats. Therefore, machine learning’s ability to merge structured and unstructured data plays an important role in the healthcare industry.
2. Continuous learning and self-adjusting model
Machine learning in healthcare facilitates continuous learning, with models adapting to new data for sustained healthcare progress. Thanks to this feature, machine learning can increase treatment efficiency over time, with knowledge updated continuously to keep up with changes in pathology, drug resistance or new equipment. Besides, this also helps to save long-term costs.
3. Predictive analytics and diagnosis support
Predictive analytics and diagnosis support are also essential features of machine learning in healthcare. It helps to predict the risk of hospital readmission, post-surgical complications, or disease outbreaks based on clinical and social variables. Also, machine learning in healthcare can identify abnormalities in X-rays, CT, MRI images; detect lung nodules, retinal lesions, tumors, etc. with high sensitivity.
Thanks to its exceptional features, machine learning is making the healthcare industry more proactive and data-centric, significantly improving outcomes for patients and providers. More benefits of machine learning in healthcare will be discussed in the following parts of this blog post.
II. Machine Learning vs AI in healthcare: Key differences
While frequently used as synonyms, ‘Artificial Intelligence’ (AI) and ‘Machine Learning’ are actually different concepts. Let’s find some key differences between AI and machine learning in healthcare with Adamo Software!
1. General concept
AI employs diverse technologies, from simple rules to complex neural networks, to create intelligent systems that can reason, solve problems, and understand natural language. It refers to any computer system simulating human thinking, learning, or behavior. Whereas, machine learning is just a branch of AI. It offers tools and techniques for building intelligent systems, emphasizing algorithms that enable computers to learn and improve from data without explicit programming.
2. Degree of automation and ability to learn
Degree of automation and the ability to learn are also the core differences between machine learning and AI. Traditional AI is often rule-based systems that are ideal for simple, predictable scenarios. They are easily controlled by pre-programmed rules. However, this limits traditional AI’s adaptability, making it quickly obsolete as data or pathological variants change.
Conversely, machine learning is designed to learn from real data. Machine learning in healthcare learns from previous existing cases to make predictions or classify new cases. For example, a machine learning model can read various CT scans to automatically detect signs of lung cancer with increasing accuracy over time. Besides, thanks to the ability to learn continuously, machine learning in healthcare can adapt to changes such as epidemiology, new treatment trends, or even changes in equipment.
3. Application in healthcare industry
AI applications include voice recognition in clinics, intelligent surgical robots, and virtual patient care assistants. Whereas, machine learning in healthcare predicts disease risk, classifies diagnostic images, detects physiological abnormalities, and optimizes drug delivery. Besides, while AI is often used in broader care or behavior applications, healthcare ML is typically integrated into systems like EMR, PACS, wearables, and medical IoT devices.
When you understand the distinctive characteristics between AI and machine learning in healthcare, you will know how to adopt them effectively. For instance, AI might design intricate diagnostic systems, while healthcare ML analyzes patient data to predict outcomes and tailor treatments.
III. Benefits of Machine Learning in Healthcare
By adopting machine learning in healthcare, you can tackle many persistent challenges. Besides, thanks to this innovation, patient outcomes can be improved. It enables healthcare providers to deliver faster, more accurate, and more cost-effective services. Its extensive capabilities, from personalized treatments to predictive analytics, are transforming the industry.
Machine learning optimizes healthcare resources, boosts diagnostic accuracy, and streamlines workflows, leading to better patient experiences. Let’s find out some exceptional benefits of machine learning in healthcare with Adamo Software!
Automated routine tasks
Machine learning in healthcare stands out for its ability to automate repetitive administrative and technical tasks. This helps healthcare staff save time and reduce their workload. It also helps healthcare facilities save operational costs. Machine learning models quickly and accurately process vast amounts of data, such as EMRs, ICD codes, test results, and medical images.
For example, a machine learning system can prioritize X-rays with likely abnormalities for physician review, removing the need for manual checks. Similarly, ML medical coding tools can similarly auto-suggest diagnostic codes from clinical records, cutting down administrative work. Healthcare ML automates these tasks, increasing efficiency and reducing human error. Additionally, it allows doctors and nurses to concentrate on specialized tasks like diagnosis and patient care.
Making more accurate decisions
ML helps clinicians streamline diagnostics and make data-driven decisions, improving patient outcomes by detecting patterns in vast healthcare data. Healthcare ML accurately forecasts disease risk, progression, or treatment response using historical case data. Besides, machine learning also helps personalize treatment by examining how genetics, imaging, and treatment efficacy relate.
Enhanced patient experience
Machine learning provides a personalized and convenient experience for patients throughout their treatment. It helps design personalized treatment paths by analyzing behavior, medical history, and real-time data. The treatment includes optimal appointments, drug dosages, and early relapse warnings.
Healthcare organizations can enhance patient experience by streamlining access to basic services with machine learning chatbots and virtual assistants. The healthcare apps that use machine learning can remind patients to take their medications on time. Besides, they monitor symptoms via wearable sensors or automatically answer common questions via medical chatbots. Additionally, machine learning in healthcare can reduce wait times by optimizing appointment schedules, allocating resources efficiently, and prioritizing patients early.
Accelerated innovation
By using machine learning, the healthcare industry can have the drive to innovate, especially in drug researching, diagnostics and development. Machine learning’s rapid data processing significantly accelerates the transition from research to practical application. Machine learning optimizes drug discovery and development, cutting pharmaceutical time-to-market and research costs. Thanks to the support of machine learning in healthcare, modern medicine can gain faster, more precise, and more personalized innovation.
Extended access to healthcare
Machine learning in healthcare’s efficiencies enables healthcare organizations to help more patients without compromising care quality. It helps to expand healthcare services to remote areas or places with shortages of skilled healthcare workers. Remote diagnostic tools, smart medical chatbots, and automated health monitoring allow patients to access advice and monitoring without frequent facility visits.
Additionally, machine learning in healthcare plays an important role during epidemics or disasters. It helps to provide medical support in case movement is restricted. Symptom tracking, epidemiological forecasting, and infection risk warnings enable quicker responses from authorities and hospitals.
Reduced risks
By using machine learning in healthcare, doctors can detect serious illnesses early. It also helps to reduce robot-assisted surgery risks and quickly identify high-risk patients. Machine learning can significantly reduce healthcare risks, from diagnostic errors to treatment complications and patient management issues. Thanks to comprehensive and accurate data analysis, machine learning can support timely medical decisions.
Read more: Computer Vision in Healthcare: What it is, real hospital use-cases, and limitations
Ready to Outsource?
Get top-tier IT talent without the hassle. Contact us now!
Refined data governance
Machine learning algorithms help healthcare organizations find inconsistencies, clean, and validate diverse datasets. Machine learning plays a key role in managing, processing, and protecting complex data sources effectively. Patient data can be diverse from electronic health records (EMRs), medical images, test results to data from smart wearable devices. Thus, machine learning in healthcare is needed to arrange these data so that doctors can easily follow.
Better public health monitoring and epidemic prediction
For public health, ML is crucial in identifying patterns and trends that shape policy. Predictive models use multi-source data, such as hospital records, social media, environmental sensors, etc., to forecast disease outbreaks and epidemics. It enables authorities to allocate resources effectively and implement preventative measures sooner.
IV. Challenges of ML in Healthcare
Despite its significant advantages, machine learning in healthcare also faces diverse challenges. They emphasize the complexity of integrating technology into a field prioritizing patient safety, data integrity, and regulatory adherence. Let Adamo Software discuss these obstacles in detail.
Data quality and availability
Inconsistent data quality and restricted data access are major barriers to implementing machine learning in healthcare. Diverse healthcare data is often unstandardized and fragmented. Unstructured data, such as clinical notes or handwritten prescriptions, also hinders ML model training. Data quality can also be affected by data entry errors, missing information, or inconsistencies between sources. Missing or inaccurate data reduces prediction accuracy and reliability.
Regulatory compliance
As a highly regulated industry, healthcare demands adherence to standards such as HIPAA (Health Insurance Portability and Accountability Act) and GDPR (General Data Protection Regulation). Ensuring ML tools adhere to these frameworks makes its deployment become more complex. While technology is more innovative, constantly updating regulations and technology transparency requirements remain a major challenge for developing machine learning in healthcare.
Algorithm bias
Bias in machine learning algorithms remains a major concern. Biased predictions can result from training models on non-diverse or unrepresentative datasets, affecting certain patient groups and hindering equitable care. The reason is that the datasets are not representative of the entire population. For example, they may lack data from the elderly, pregnant women, or ethnic minorities.
Ethical concerns
Using machine learning in healthcare raises concerns about patient privacy, informed consent, and decision transparency. Personal health data often contains highly detailed and private information like medical history, test results, diagnostic imaging, and genetic information. Patients may be worried that their data is used without their permission for improper purposes. Clear guidelines are essential to address these issues and build trust.
Integration with existing systems
Healthcare facilities commonly use a variety of patient management software, medical devices, and data storage systems with differing data standards or interoperability. Thus, integration with existing systems is also a challenge of adopting machine learning in healthcare.
Effective ML models rely on seamless, accurate, real-time data sharing during integration. However, fragmented and unstandardized healthcare data make this difficult. Additionally, outdated existing models might not technically support modern ML solutions, leading to costly and lengthy upgrades or replacements.
Cost and resource constraints
Implementing machine learning in healthcare often requires substantial investment in technology, infrastructure, and talent. This may be a significant problem for small healthcare providers or those in resource-limited settings. They often lack the financial resources and technical expertise to build, operate, and continuously update complex ML systems.
Additionally, training healthcare workers to use ML tools effectively requires significant time and resources. Without proper preparation, ML solutions can be misused or wasted.
V. Best use cases of Machine Learning in Healthcare
Machine learning is helping research institutions, companies, and startups innovate and create transformative solutions across healthcare, spanning diagnostics to personalized medicine. Let’s find some best use cases of machine learning in healthcare with Adamo Software!
Research
In a lab, ML can help researchers understand disease mechanisms and improve hypothesis testing. Algorithms analyze advanced datasets to simulate disease progression, providing researchers insights into how conditions develop and respond to treatment. This is especially valuable for diseases like cancer, where predicting patient response to specific drugs can lead to more personalized treatment strategies.
Disease Diagnosis and Prediction
Disease diagnosis and prediction is also among the best use cases of machine learning in healthcare. Thanks to their ability to process large volumes of data, medical images, lab tests, or genetic data, adopting machine learning in healthcare helps to identify abnormalities.
For example, in radiology, machine learning models are trained to examine X-rays, MRIs, and CT scans, detecting anomalies like tumors or lesions with remarkable precision. These models are vital for early diagnosis in conditions such as cancer or cardiovascular disease, where early detection is critical for successful treatment and patient survival.
In addition to assisting doctors in the diagnosis process, ML also helps predict the possibility of disease recurrence or the risk of re-hospitalization, thereby allowing early intervention and minimizing risks for patients. Thanks to continuous learning, these models become more accurate, helping to improve the quality of care and optimize treatment processes.
Personalized medicine and treatment plans
By using machine learning in healthcare, healthcare facilities can personalize patient treatment instead of applying a general solution to everyone. By analyzing multidimensional data such as medical records, genotype, lifestyle, drug response, and test results, ML can determine the optimal treatment for each individual.
This is especially valuable in oncology, where treatment effectiveness differs widely between patients. Machine learning algorithms can analyze tumor genetic data to recommend more effective chemotherapy or targeted therapy regimens, increasing response rates and reducing side effects.
Drug discovery and development
Machine learning in healthcare aids drug development by accurately identifying potential new drugs and predicting their safety and effectiveness. It analyzes complex biological data like genetics, protein structures, and molecular interactions, to predict how compounds interact with target proteins and enzymes.
This model predicts how chemical changes affect drug performance for patient safety, also increasing clinical trial success. Besides, machine learning in healthcare also analyzes past clinical trial data to predict new drug performance and risks while enhancing trial design for efficiency by identifying patient populations, dosing, and side effects.
VI. Embrace digital transformation in healthcare software development with Adamo Software
As one of the leading technology companies in Vietnam, with many years of experience working in the healthcare field, Adamo Software can help you digitize your healthcare facilities system with the use of machine learning in healthcare. Let’s see how Adamo Software can help.
Customizing medical software solutions according to actual needs
As each healthcare organization has its own operating procedures and characteristics, Adamo Software focuses on developing custom healthcare software that fits each partner’s goals and operating model. We offer solutions in tailoring Electronic medical record management systems (EMR/EHR), patient portal, disease monitoring and management tools, etc. Thus, healthcare facilities can deploy flexible, effective and practical technology systems.
Integrating machine learning in healthcare
Adamo helps healthcare organizations apply Machine Learning to care and operations processes. They include supporting medical image analysis and diagnosis, predicting disease risk and complications, and suggesting personalized treatment regimens. Besides, we also support analyzing patient data to optimize care.
Ensuring security and compliance with medical regulations
With experience in deploying software globally, Adamo Software concentrates on medical data security. Our solutions strictly comply with standards and regulations like HIPAA, GDPR, etc. Thanks to this, healthcare facilities can deploy the technology without worrying about legal violations.
With technological capabilities and extensive experience in the healthcare industry, Adamo Software can be your ideal partner to help you maximize the potential of machine learning and new technologies in modern healthcare. Still hesitating whether to use Adamo’s service? Let’s contact us for more detailed information!